Students apply mathematical know-how to solve real-world logistical problems
By Katie Neitz
At the heart of every school’s academic scheduling, every Google Maps route calculation, and every Amazon Prime delivery order are mathematical algorithms.
Math is the universal language behind many everyday-life operations. It’s a tool that can provide fast, reliable solutions to a range of complicated problems, whether the goal is to schedule classes appropriately to prevent a professor from being double-booked or to ensure a package arrives on time.
In his course Math 306: Operational Research, Jonathan Bloom, associate professor of mathematics, aims to teach students how companies and organizations rely on math to operate smoothly and efficiently.
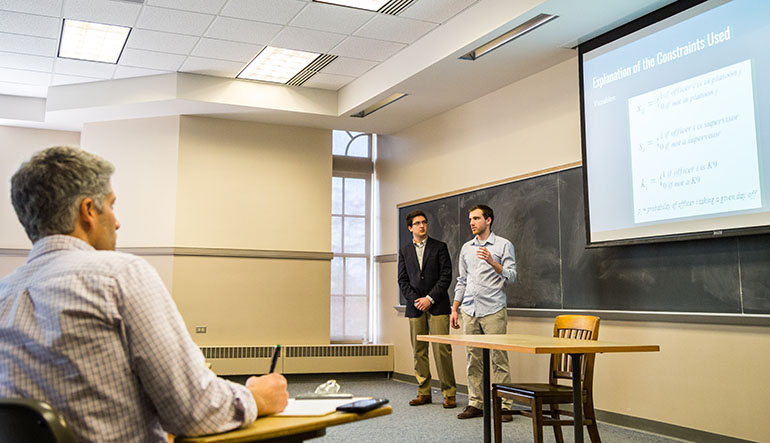
Students in Jonathan Bloom’s Operations Research class delivered their final presentations to their classmates and clients.
To do that, Bloom puts his students in the role of consultants—giving them the opportunity to apply their mathematical knowledge to solve real-world problems posed by local community businesses and organizations.
This spring, for the third iteration of the class, the clients were: the Easton Public Market Farmstand, the Easton Police Department, and Lafayette’s own Office of Residence Life.
Bloom begins the semester introducing students to linear and integer programming, which are fundamental algorithms used to optimize real-world problems.
A few weeks in, he shifts the format of the class, pairing up small groups of students with the clients. Students meet with their clients throughout the semester with the goal of presenting the clients with mathematical models that give them valuable insight and information, enabling them to make more informed decisions about their operations.
“It’s more than just the math,” Bloom says. “The students are expected to act like professional consultants and interact with a client. The client likely knows nothing about linear programming. And the students likely know nothing about the client’s work. So the students need to be able to ask the right questions and communicate clearly.”
In the real world, Bloom says, data collection can be messy and murky. And that’s the point. “I’m trying to give them an experience they couldn’t get with a traditional homework assignment,” he says.
Students say he succeeded.
“It was really a valuable experience to be able collect the data and not just use data that was provided to me,” says Corey Chelak ’19, who is majoring in mathematics and economics. “We struggled in the beginning. We had to get together with our client and then meet together as a group a lot to really think through it. I learned a lot in that process, and it made it more rewarding in the end.”
Here is an overview of the clients’ problems and the insights Bloom’s students provided.
The Client: Easton Public Market Farmstand
The Problem: Christopher Wolf, Farmstand operations manager, has lots of options for where to buy tomatoes. He knows his customers prefer locally grown and organic produce. But they also want his prices to be affordable. And he still needs to make a profit. So Bloom’s students set out to create an algorithm that would help Wolf be able to determine the optimal choice of where to purchase tomatoes for the stand—year-round—taking all of these variables into consideration.
The Solution: Students provided Wolf with a model, implemented in Excel, that gives him a simplified and holistic view of all the different factors that go into his purchasing decisions. The control panel created for him enables him to sort the data so he can see what the best overall combination of factors is to consider when purchasing tomatoes. Wolf can input current prices, minimum orders, supply, demand, and “level of quality,” and the program students devised will automatically determine the optimal buying decision. He can adjust it as needed to take into consideration changing factors like seasonality and tomato varieties. The hope is that he’ll be able to apply the same model to other types of produce so it informs all of his purchasing decisions.
The Feedback: “I’m impressed by the professionalism of the students; their insight is really valuable,” Wolf says. “This tool enables me to easily see which farms I should be ordering from in order to get the best quality and also make a profit. This allows me to make the best business decision.”
The Client: Office of Residence Life
The Problem: Residence advisers (RAs) need to do nightly rounds, in which they walk through the residence halls they oversee. With the current assignments, one RA’s rounds takes 28 minutes to complete while another RA’s rounds takes only 11 minutes, a difference of 17 minutes. RAs assigned to the more time-consuming routes have expressed frustration to Julie Mule, associate director of residence life. Residence Life has utilized several models over the years in attempt to keep staff size and duty areas close in range to each other. Mule was eager to collaborate with Bloom’s students to get mathematical analysis to see if duty areas could be reconfigured differently so that the workload would be divided more equitably.
The Solution: Students measured distances between all the different residence halls on campus and factored in the average walking speed of the RAs. Students devised three different possible ways that Mule could cluster the residence halls to reduce the time between the longest and shortest rounds. Although it wasn’t possible to make all the rounds exactly equal, one model the students proposed would reduce the difference between the longest and shortest routes to 11.5 minutes. That’s a significant difference from the current disparity of 17 minutes.
The Feedback: “It’s so valuable to have this information and to see the various options for routes,” Mule says. “I think that model 3 has potential because it really cuts down the difference between rounds. That is something for us to look at further and consider. This is also valuable because in the future if RAs ask why the rounds are not equal, I can explain that they are as equal as possible—and we have the mathematical model to prove it.”
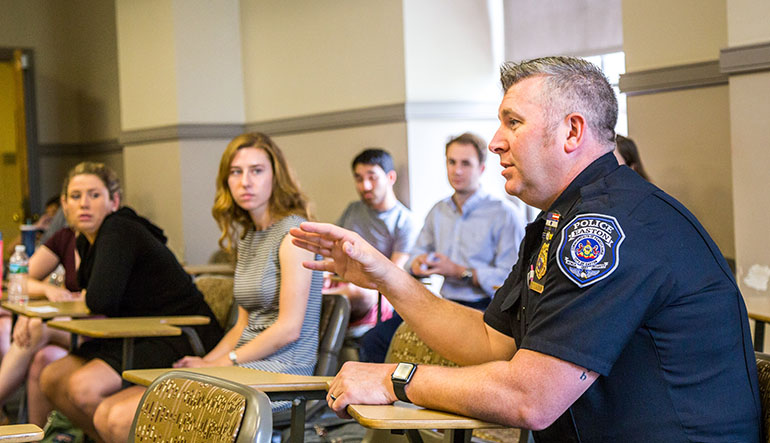
Lt. Robert Weber gives feedback to the students following their presentation.
The Client: Easton Police Department
The Problem: Scheduling officers’ shifts is complicated. Each day shift and night shift need to be covered by a certain number of officers and a supervisor. That might seem straightforward. But consider that officers are required to go to monthly training sessions (which take them off their shifts), have different types of specialties (SWAT, bicycle, K-9), have vacation/sick/personal time, have an irregular schedule (officers work three days one week, four days the next). Plus, there are special events in the city that may need extra coverage. And there’s the bonus factor that certain officers want to work together on the same squads. “It’s tough to coordinate it all,” says Lt. Robert Weber. “We can’t be short-staffed. At the same time, we also want a good working environment for our officers. We want them to be on squads with officers they have strong relationships with. You work better and serve your community better when those factors are taken into consideration.”
The Solution: Two groups of students tackled this project. One group looked at the probability of an officer taking time off and then optimized the squads so it reduced the likelihood of too many officers taking time off at the same time. The other group tried to accommodate officer preference for whom they wanted to work with. They approached it from a “happiness” perspective, working to cluster officers into squads that maximized their on-the-job satisfaction. Their model was built with a “penalty factor,” which accounted for two officers who wanted to work together but were also likely to take the same day off work. The groups developed models using data available, but they ran into some limitations and constraints. For instance, labor contract rules and officers’ seniority play major roles in scheduling—and can’t be altered. And due to privacy issues, the students couldn’t access data that could be helpful in gauging officers’ probability of taking time off on certain days, like age or marital status. The students worked within these confines to develop recommendations based on factors they could control. And in doing so, they laid the groundwork for what could be an ongoing project for next year’s class.
The Feedback: Although there wasn’t an easy-to-implement immediate solution to this, Weber said it was a valuable experience, and he’d like to continue to work with Bloom’s students in the future. “I think it’s a great opportunity to have outreach with kids on campus,” Weber says. “They get to interact with us on a professional level. It was fun. They get to ask questions and learn what it’s like to function in a profession like ours. So we’re not just enforcement. I think they have a better understanding of what we do. If other areas want to do projects with us, we are more than happy to participate.”